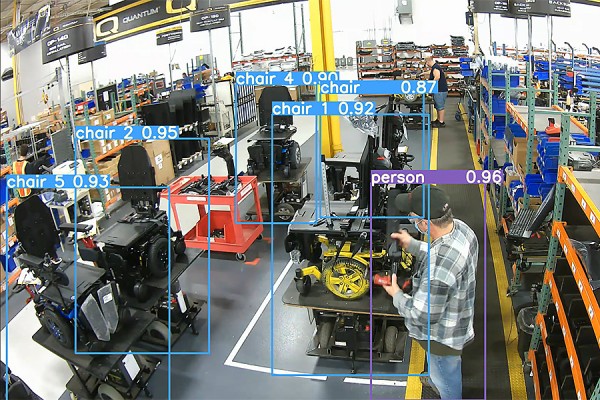
Two UWindsor engineering researchers have received more than $715,000 in federal funding to bring cutting-edge artificial intelligence to the manufacturing floor.
Professors Jonathan Wu and Afshin Rahimi say they can mitigate human error and maximize productivity in manufacturing plants through advanced computer vision.
“Human errors were the major driver behind $22.1 billion in vehicle recalls in 2016,” says Dr. Wu, a former Canada Research Chair in Automotive Sensor and Information Systems.
He and Dr. Rahimi aim to create a smart production assistant that will help manufacturing plant operators gain unprecedented visibility into their manual production operations, allowing them to optimize their worker efficiency while maximizing productivity. They will achieve this by automating data generation using computer vision, converting raw data into useable information, visualizing information using common business intelligence methodologies and prediction of future.
The professors have received $717,450 of support from the Mitacs Accelerate program and additional support from Smart Computing for Innovation (SOSCIP) in partnership with i-5O, an early stage Silicon Valley based start-up that has developed a proprietary computer vision powered digital twin to help manufacturers track, measure, and improve their manual production processes. Headquartered in San Francisco with operations in Toronto and Windsor, the company works with large Fortune 500 manufacturers in North America and Asia.
Khizer Hayat, chief innovation officer of i-5O, says its collaboration with Wu and Rahimi will bring the latest in artificial intelligence for improving human performance to the manufacturing industry.
“Thanks to their expertise, our collaboration has created a cutting-edge artificial intelligence product that delivers double digit percentage increases in human performance to our clients,” Hayat says.
Rahimi’s team focus on three major components of the project, including data extraction and processing for object detection and classification using computer vision, deep learning, and neural networks; hardware design and optimization for fast data-processing on-site; and data visualization for information translation and user interactions.
Wu’s team will develop a computer vision and deep learning based temporal action detection model and apply it to the video collected from manufacturing floors to obtain the start time and end time of each process in the operation.
More than 70 per cent of tasks in manufacturing are still manual, therefore, more than 75 per cent of the variation in manufacturing comes from human beings,” Rahimi says. “Currently, when plant operators want to gain an understanding of their manual processes, they send out their highly-paid industrial engineers to run time studies.”
Automated inspection monitoring can help plant operators save both money and time, Rahimi adds.
Cost savings can also occur in future phases of the project by informed planning from forecasted trends in production line performance.
—Kristie Pearce