Friday, March 24, 2023 - 10:00 to 11:30
SCHOOL OF COMPUTER SCIENCE
The School of Computer Science is pleased to present…
MSc Thesis Defense by: Raghib Barkat Muhib
Date: Friday March 24th, 2023
Time: 10:00 AM - 11.30 AM
Location: Essex Hall, Room 122
Reminders: 1. Two-part attendance mandatory (sign-in sheet, QR Code)
Reminders: 1. Two-part attendance mandatory (sign-in sheet, QR Code)
2. Arrive 5-10 minutes prior to event starting - LATECOMERS WILL NOT BE ADMITTED. Note that due to demand, if the room has reached capacity, even if you are "early" admission is not guaranteed.
3. Please be respectful of the presenter by NOT knocking on the door for admittance once the door has been closed whether the presentation has begun or not (If the room is at capacity, overflow is not permitted (ie. sitting on floors) as this is a violation of the Fire Safety code).
4. Be respectful of the decision of the advisor/host of the event if you are not given admittance. The School of Computer Science has numerous events occurring in the near future.
Abstract:
Vehicle classification is an essential part of intelligent transportation systems (ITS). This work proposes a model based on transfer learning. This combines data augmentation for the recognition and classification of local vehicle classes in Canada. Our study is inspired from contemporary deep learning (DL) achievements in image classification. The well-known Stanford AI of Vehicles Dataset, which contains 16185 pictures, is used for this. The images in this Dataset are divided into 196 types of various vehicles. To increase performance additional classification blocks are added to the residual network (ResNet50)-based model. In this case, vehicle type details are automatically extracted and classified. A number of measures like accuracy, precision, recall, etc. are used during the analysis to evaluate the results. The proposed model exhibits increasing accuracy despite the vehicles' shifting physical characteristics. In comparison to the current baseline method and the two pre-trained DL systems, AlexNet and VGG-16, our suggested method outperforms them all. The suggested ResNet50 model achieves an accuracy of 90.07% in the classification of native vehicle types. We have also compared this by running VGG-16 where we are getting an accuracy of 82.5%. Along with this Vehicle classification, we have implemented number plate detection and smart vehicle counter systems which all together makes our transport system better than ever before.
Keywords: Vehicle type classification, Deep learning, Convolutional Neural Network, Transfer learning, ResNet-50, VGG-16 , ANPR, OpenCV, EasyOCR.
MSc Thesis Committee:
Internal Reader: Dr. Dan Wu
External Reader: Dr. Esam Abdel-Raheem
Advisor: Dr. Imran Ahmad
Chair: Dr. Boubakeur Boufama
MSc Thesis Defense Announcement 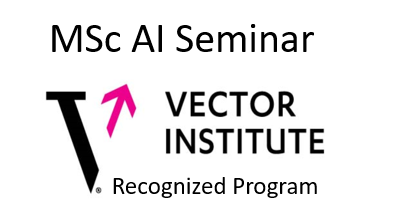
5113 Lambton Tower 401 Sunset Ave. Windsor ON, N9B 3P4 (519) 253-3000 Ext. 3716 csgradinfo@uwindsor.ca