Monday, April 10, 2023 - 10:00 to 12:00
SCHOOL OF COMPUTER SCIENCE
The School of Computer Science is pleased to present…
MSc Thesis Defense by: Laveen Bhatia
Date: Monday April 10th 2023
Time: 10:00 am – 12:00pm
Location: Essex Hall, Room 122
Reminders: 1. Two-part attendance mandatory (sign-in sheet, QR Code) 2. Arrive 5-10 minutes prior to event starting - LATECOMERS WILL NOT BE ADMITTED. Note that due to demand, if the room has reached capacity, even if you are "early" admission is not guaranteed. 3. Please be respectful of the presenter by NOT knocking on the door for admittance once the door has been closed whether the presentation has begun or not (If the room is at capacity, overflow is not permitted (ie. sitting on floors) as this is a violation of the Fire Safety code). 4. Be respectful of the decision of the advisor/host of the event if you are not given admittance. The School of Computer Science has numerous events occurring soon.
Abstract:
Deep Learning is one of the most evolutionary concept in the field of Artificial Intelligence, allowing us to train a Machine Learning model for almost any type of problem using any type of data. Federated Learning (FL) is a type of distributed Deep Learning framework, in which the model is trained locally on each device, and only the trained gradients, also known as “local updates”, are sent to a central server which aggregates them and creates a global model. This helps in preserving the data privacy of the user as the local data never leaves the local device. It has many applications in field of healthcare, supply chain, finance and many more. However, due to its heavy reliance on a central server, it poses many issues, such as communication bottleneck, a single point of failure and trust issues due to lack of transparency. Another major concern in Federated Learning is ensuring the data quality of trained data. Since there is no control over the training data, FL models tend to be highly susceptible to model poisoning attacks. To address these issues, we propose a decentralized approach using blockchain to create a FL framework. Blockchain provides a decentralized (no reliance on central server), transparent, immutable, traceable, and a trustless environment. We use miners to validate every local model by running it against a secret testing dataset and checking its accuracy. This is done using a smart contract. The local model will be aggregated with the global model only if it passes a preset accuracy threshold. We test our proposed method on a Brain Tumor Classification dataset from Kaggle, comprised of 7000 MRI images divided into 2 classes (Tumor / No Tumor). Our results show that our method performs up to 68% better than the original Federated Learning approach on the testing data.
Keywords: Federated Learning, Blockchain, Decentralized Federated Learning, Decentralized
Data Evaluation, Smart Contracts
MSc Thesis Committee:
Internal Reader: Dr. Pooya Moradian Zadeh
External Reader: Dr. Mohammad Hassanzadeh
Advisor: Dr. Saeed Samet
Chair: Dr. Ahmad Biniaz
MSc Thesis Defense Announcement 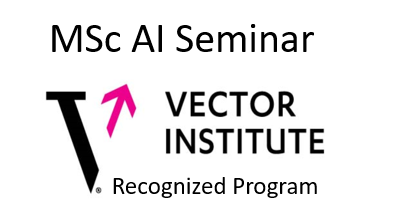
5113 Lambton Tower 401 Sunset Ave. Windsor ON, N9B 3P4 (519) 253-3000 Ext. 3716 csgradinfo@uwindsor.ca